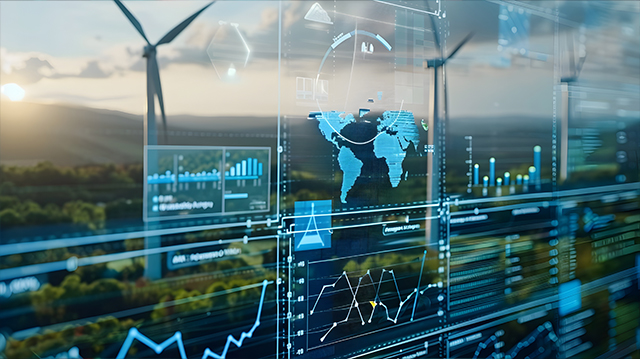
AI-based applications in decentralised electricity systems
- Project team:
- Thematic area:
- Topic initiative:
Ausschuss für Bildung, Forschung und Technikfolgenabschätzung
- Analytical approach:
TA-Projekt
- Startdate:
September 2024
- Enddate:
2026
sprungmarken_marker_4448
Background and central aspects of the topic
Growing shares of renewable energy lead to increasingly decentralised electricity systems that require a more flexible control of a large number of small-scale generators, storage facilities, and consumers. In this context, AI-based applications are developed and tested to allow for more flexibility and increase the resilience of the electricity system. This includes particularly machine learning methods (techniques, algorithms, and models) such as artificial neural networks, random forests, or clustering. These methods can be used to analyse large amounts of data more efficiently in order to improve forecasts and optimise the control of decentralised systems. They can be applied in very diverse areas such as predictive maintenance, anomaly detection, load and feed-in forecasts, or the control of flexible loads. Many AI methods are already used in the electricity system. However, they are expected to become more important in the coming years due to increasing digitalisation and, in particular, the planned expansion of the smart metering infrastructure.
Apart from the opportunities, there are also risks associated with this development. In the TAB Foresight Report, trends related to digitalisation were consistently classified as factors for increased system vulnerability. A key issue is cybersecurity, including both (intentional) cyber attacks and (unintentional) failures, e.g. due to software errors. In addition, economic and geopolitical dependencies are important considerations, as well as concerns for consumer privacy, data security and empowerment. The latter issues become more pressing the more AI-based applications are used in private households.
Objective and approach
The aim of this TA project is to gain insights into the current and future use of AI in decentralised electricity systems, to assess the opportunities, and identify risks associated with AI-based applications.
The study is limited to the following areas:
- AI-based applications related to consumers or prosumers that aim to integrate small and medium-sized generation and storage facilities more efficiently and flexibly into the grid, and to match electricity demand to the availability of renewable supply. Examples include demand response programmes, the aggregation of small-scale generation and storage into virtual power plants, as well as vehicle-to-grid, vehicle-to-home, and smart charging applications.
- AI-based applications at the neighbourhood or community level that aim to match demand to the availability of renewable supply and thus maximise the local use of renewable electricity. Examples include shared battery storage (neighbourhood-scale batteries) or photovoltaic systems.
- AI-based applications at the distribution grid level that aim to deal with systemic risks and increase resilience. In particular, this involves forecasting potential damage and outages caused by extreme weather events and identifying anomalies to detect and protect against cyber-attacks.
The project consists of two phases. In the first phase, we will provide an overview of current applications of AI methods in electricity systems and assess opportunities and risks of applications in the three areas described above. In the second phase, we will discuss in detail selected applications that have been identified as particularly promising during phase 1.
Project status
As part of the TA project an expert opinion has been commissioned that provides an overview of AI methods and AI-based applications for decentralised electricity systems at the consumer/prosumer, neighbourhood and distribution network level and assesses their potential.